In a previous article, Conquering Analytics: Collection, Validation, Enhancement, Accessibility, I spoke about how to handle data in a complex, general, analytics system. In this article, I am going to…
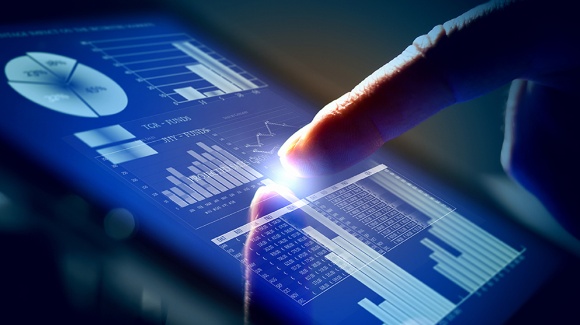
Conquering Healthcare Analytics: Consolidate, Prepare, De-identify and Analyze
In a previous article, Conquering Analytics: Collection, Validation, Enhancement, Accessibility, I spoke about how to handle data in a complex, general, analytics system. In this article, I am going to assume that you have read my previous article and in a little more specific of a use case, I will talk a bit about taking that mindset and handling healthcare analytics.
I’ve had the privilege of working with all kinds of data in my career, none more challenging (and rewarding) than working with healthcare data. Context is key to how you structure data for success. Let’s look at the types of data that are typical in healthcare.
- Patient Data
- Clinical Data
- Cost Data
- Claims Data
Each of these data sets has unique characteristics that should be considered when you are working with it. A single data set is useful, but combining from all four(4) of these types is powerful. The important consideration, however, is that some of this data, particularly patient data, contains “Protected Health Information” or PHI data that must be handled appropriately. HHS has some guidelines for HIPAA that you should familiarize yourself with.
Consolidate and normalize data
When you are working with Electronic Medical Record (EMR) data, if you know one thing then you know how little the each looks like the next. The EMR systems don’t communicate nor do they store data in a consistent way. Any type of data can be collected and normalized that into a consistent and workable healthcare standard. What a patient is in one EMR or analytics system, becomes a standardized patient record. That isn’t always advantageous for analytics. This is a consistent problem when working with healthcare data. I cannot stress this enough…
Do not assume that normalization is the solution to analytics. It is the first step in the preparation of data.
This disparate data can be made available in a more relational way so you can query and model across all types of healthcare resources.
Protecting patient data
First and foremost, you need to protect any patients that may be part of the data. Healthcare analytics providers have a singular responsibility to make sure the rights of those people are protected. This is done through a few processes. I prefer to handle this during the validation stage after the data has been collected. Some people may choose to protect the data during the collection stage.
The main goal is to anonymize or de-identify the data so that no identifiable data can be discerned from the full data set but all the actual records that will be processed will remain. There are some interesting challenges that present themselves when doing this. I won’t go through all of the HIPAA rules here but generally, after de-identification happens, the data should be protected from re-identification (as much as possible). Think specifically about populations, both at the geographic level and the provider level.
Take this example:
Provider: You have a physician who only has 100 patients (patient “population”). A patient has some identifiable characteristics that if known and combined with other general data you could infer their identity. Inference IS identification so take care not to misunderstand that fact.
Geographic: A patient has some identifiable characteristics and you know they are in a certain location. That specific location is in another set of data that says, there are 100 patients in the area from three(3) providers. If enough general data is given (characteristics), you could infer the patient specifics because the list of patients for a given area and a given physician would make the patient population so small you could make an educated guess.
The takeaway from this is that just because you take a person’s PHI data out, doesn’t necessarily make you compliant. HIPAA compliance also has some additional safeguards you should take into account. Look specifically at the geographic rules for postal code and IP translation.
So, you have the data de-identified, now what?
Purpose
The next thing to decide is the purpose of the analysis. What are you trying to accomplish with the data?
- research analysis
- operational analysis
- segmentation for risk analysis
- segmentation for creating populations
When you determine what you want to accomplish, you can set your data up in a number of ways. You should always consider building standard research models into your workflow. The underlying correlations almost always expose interesting storylines. So, here is where most healthcare analytics go wrong and I get the most push back. I almost always get the following question:
“But John, don’t you need to know the questions you want to ask?”
Short answer, no. Reporting answers questions, analytics tells stories that lead to more questions (which should ALWAYS be your goal). Read my article on Analytics is storytelling, not reporting for more specifics. If you structure your data to be able to answer any questions, you will always be in a position for success. Most commercial industries have been doing this for the last few years and have been very successful in taking data and creating actions that lead to successful business outcomes. There is nothing that is preventing the healthcare industry from taking the same path except for status quo.
Vanity metrics & comparing data
You should always take into account the “vanity metrics” that go along with the data. What is a vanity metric? A vanity metric is any metric that is based on volume but holds no value on its own. A simple example of one of these in healthcare might be “Total # of Patients”. If you are in a practice and you want to know this number, you would have to know some other data before this can provide business value. So, let’s look at this example specifically.
If I tell you that you have 525 patients in your practice right now, would you say that is good or bad? Well, some smaller providers might say good, while some larger providers might say bad. Perspective and context is key to understand the data. You can also think of it as having a need for some kind of comparison. Examples of this context may be:
- How many patients did I have last month (month over month change)
- What other initiatives did we push to gain patients during this month
- How many patients are active? What % of these patients did we actually see in the office this month?
You start to see that time and general status is important but that number on it’s own holds no business value. It makes people feel good but doesn’t kick anything into action.
Analyze and modeling
Once all the data is sourced, protected and prepared, now the fun begins. The normalized or in some cases, de-normalized data can now be used to run standard algorithms to find patterns and correlations, or more advanced predictive models can be run to answer many questions regarding population health and/or risk management. If you structure the data right, you can also segment out populations for individual assessment and compare segments. Sometimes you might just need standard trend or regression analysis but all of this is now available.
It is exciting to see what is next for healthcare analytics, specifically in the managed care space. Hit me up with any questions, would love to chat. john@slingspace.com