“We have a bad data team.”
“We can’t rely on our data.”
I sometimes hear people in the industry say this. I usually just focus on solving the problem. But, I’ve been thinking about this for a while. I think to myself, “What is the real issue here?”
Business leaders have been tricked into thinking that data is the answer to everything. Reinforced every day by a new vendor claiming to solve all the issues but there are a couple of core things that play into these feelings.
First, most data problems are business problems. Problems with collection, problems with instrumentation, or problems with interpretation of business context. Take this arithmetic problem. We have been trained from an early age that if you see an equation like 1+2 = 5 that the answer is wrong.
- What if i told you that it would depend on perception?
- What if 5 isn’t the problem?
- What if I told you that the number 2 is the issue and that it should be 4?
Many times, the core of our issues in data stem from something other than the answer. Amazing how most things are given the luxury of context but our industry (data/tech) is not. Many times, the excuse someone has is “I was given bad data”. Sometimes, that is the case but I would offer up a few solution paths to make sure the problem is being attacked from the right angle.
Ask yourself the following questions
- Does the metric represent the business definition (or the definition I am thinking of)?
- Is the pipeline functioning correctly?
The first question requires a conversation with your data leader to truly understand what you are asking. Do not take matters into your own hands and interpret data without that conversation happening. Data is NOT easy and no matter what you think, even the smallest misconception can lead to bad perception.
Note: This is not bad data. This is not a data quality issue.
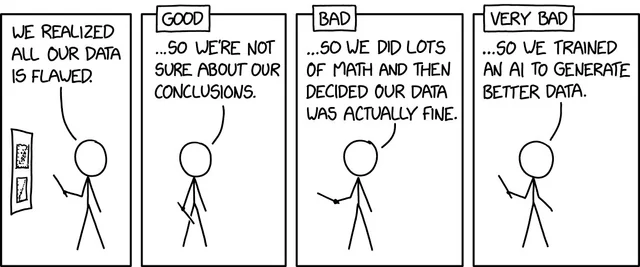
Enter Data Observability tools. With a tool that can track data flow and expectations across the entire stack, you can be sure that your data quality is 100%. Although Observability can’t help with issues of perception or misunderstandings of definition, you can guarantee your business that you have the right data.
You can be consistently wrong but not inconsistently right.
What does this mean? Basically, if I can reproduce an issue of calculation and get the same answer everytime, I can fix the issue and move forward. If I can’t explain why I get one answer that is right one day but get something different the next, people will lose confidence. Data Observability tools give you (and the business) confidence that your pipelines are solid and downstream reports are functioning ‘as expected’.
Recently, when I implemented MonteCarlo’s Data Observability platform, I immediately had confidence in our data workflows. I could see if there were anomalies in expected rows, unexpected changes in data contracts and how those issues would affect our deliverables. We could even write our own custom rules that aligned to our business needs.
As a business leader, be engaged and ask to see lineage from your data teams.
Business Leader, ask the question, how can I be sure that the data is performing as expected?
If your team can’t answer that definitely, you are missing a core piece of your infrastructure.
Data Observability
Observability is not monitoring. Don’t mistake monitoring tools for observability. There are actually three main areas that should immediately give credibility to your data team. If you have and constantly review these areas, the two statements from the beginning of the article should never come up, thus ensuring your reputation and that of your team.
- Data Definitions – The agreement of what a business metric is across the entire organization. Ex: LTV means the same for sales as it does for marketing and finance. Publish these corporate metrics.
- Data Interpretation – The Data team has a responsibility to ensure that the data definitions are represented in the aggregations. If your platform doesn’t send facts to the data team, it opens up a whole different set of problems.
- Data Observability – The end-to-end visualization of data contracts and their expected outcomes. Building a data platform without an observability tool, especially at scale, will leave too many blindspots and can guarantee multiple points of failure.
In any data pipeline, you can organize it according to the following structure.
Availability > Accessibility > Reliability
“Does it exist?” > “Is it accessible to the business?” > “Can we trust it?”
We all know there are more specifics but for simplicity, we can keep the conversation light. Data Observability tools can help to make sure that the business can trust you.
These tools are a great way to instill confidence and put the conversation back to what really matters. Creating good, reliable data solutions that generate business value. Im excited to see how this will translate into affirming the reputation of Data teams and the services they provide.