At the core of every successful analytics report, dashboard or any web app that collects analytics is planning that understands the current and future needs of the audience. Every analyst…
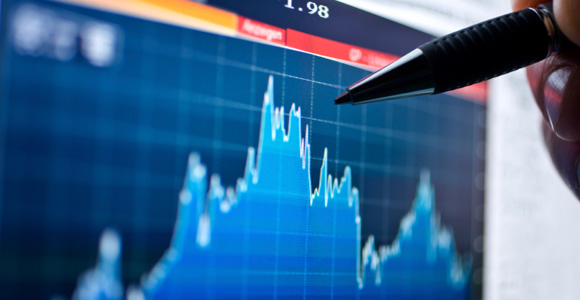
Analytics: Failure to plan is planning to fail
At the core of every successful analytics report, dashboard or any web app that collects analytics is planning that understands the current and future needs of the audience. Every analyst or person with any experience has forgotten to put analytics on their project, only to come back a week or month later and realize no data had been collected. There is no worse feeling than to know you missed out on that critical bit of information that is so important, collection of benchmark metrics for an application release.
Planning is important and every good plan should start with a checklist of needs for a release. What goes into this plan, what is required to verify that everything is what it should be? It all starts with collection of useful metrics. Is every important event being captured? Total impressions, pageviews and details around what the classifications are for each is very important. You should plan for what you need to understand before just slapping any analytics in. Will you need to classify the events. For example, think about the page, is it a product page, is it a resource or support page, to be able to classify it in a report, means you have to think at a little more specific level with the events you are capturing. Don’t rely on deriving so much that it creates more work.
Page level events should be captured as well. After all, what is the points of analytics? It should serve these major roles:
- To validate the purpose of the application
- To help you get better with every iteration
- To provide possible feedback to your consumers that what you are doing is generating the correct result
Page level events capture very specific patterns of behavior like did someone click, view or interact with your content in some way. These individual metrics drive new features, tell you which features should be depreciated and give you vital knowledge around who your audience is. If you are creative, you can build profiles around your users in order to define them and drive specific behavior based on that knowledge. Using high end data science through things like Reality Mining, you can build up behavior profiles over time that can help you to create new audience specific interactive interfaces.
You should create plans around what data collection means and understand that you can gain a deeper insight into what users want from you by analyzing longer behavior patterns.
You should have short-term and long-term plans about how to collect and use the data.
No doubt that as patterns emerge, you will create new ways to use the data but at the very least you should think about what is available and collect as much as possible. You can’t go back and get it all, so even collect information that you don’t see as benefit now.
In your plans, you should also consider cross-referencing data with other data sets. On it’s own, your data may seem relatively benign but consider how your data could bring out trends and patterns in other, more robust and generic sets of data. For example, maybe you are a retailer that has tons of brand specific information. On it’s own, it may have a finite use case but once you tie this into a larger data set like the governments release of retail data from the holiday season, you may be able to see how your sales stacked up against the national data. You may be able to spot trends that will help you make changes to your business that could increase sales.
In summary, just having analytics isn’t enough. You need to plan for what you will do with those analytics and understand what business value they will bring once the data is compiled. As an analyst, question everything and do not assume that all metrics are being captured. Define those metrics and verify the implementation and validate post release. Don’t just have an implementation plan but also a plan for post-implementation. Check back that metrics are flowing as expected on some interval (1d, 7d, 4w). You won’t be sorry.